Fifteen years ago, machine learning and AI were terms familiar mainly to specialised researchers and industry practitioners. Nowadays, AI is a topic for everyone; it’s in the newspapers, and even our dinner conversations turn to it. We’re living in an age when large language models (LLMs) can chat with us and diffusion models can generate new pictures for us. Biology is evolving to incorporate AI methods as well, employing and adjusting novel techniques for its use in tasks like protein structure prediction or genomic analysis.
This is what brought people from a variety of disciplines to the EMBO | EMBL Symposium ‘AI and biology’ held in a hybrid format from Heidelberg in March 2024. Here are just five takeaways from this dynamic event:
1. Multimodality is the new buzzword
Multimodality in machine learning means integrating diverse input data types (like different imaging techniques, expression profiles, genomic sequences or structures) into one model, and it was one of the most used words at this conference. Multimodality can help us use more diverse samples for machine learning models to learn better and provide a more holistic understanding of mechanisms in biological systems that single-mode data can’t create. One of multimodality modelling’s uses as described during the conference was in cell imaging. However, it might also be particularly useful in medicine– for example, combining genetic information with clinical data that leads to personalised treatments. Using multimodality can also lead to better-designed experiments and show us which modality carries which type of information.
2. LLMs can answer your scientific questions
We live in a new world, where LLMs like GPT or Mixtral can change how we think about classical biological or bioinformatics problems. Instead of doing classical gene set analysis by looking at resources like Gene Ontology or the Kyoto Encyclopedia of Genes and Genomes, one can use a dynamic resource. With a bit of prompt engineering, one can directly ask GPT-4 for hypotheses about common gene functions. LLMs can also assist in extracting evidence from the scientific literature to help with tasks such as drug target identification and validation. Another use may be in protein annotation, where LLMs can follow the traditional pipeline by finding the closest homologs and extracting information about them, but in a much shorter time.
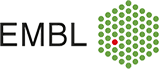
About EMBL
EMBL is Europe’s flagship laboratory for the life sciences. Established in 1974 as an intergovernmental organisation, EMBL is supported by over 20 member states. EMBL performs fundamental research in molecular biology, studying the story of life. The institute offers services to the scientific community; trains the next generation of scientists and strives to integrate the life sciences across Europe. EMBL is international, innovative and interdisciplinary. Its more than 1700 staff, from over 80 countries, operate across six sites in Barcelona (Spain), Grenoble (France), Hamburg (Germany), Heidelberg (Germany), Hinxton (UK) and Rome (Italy). EMBL scientists work in independent groups and conduct research and offer services in all areas of molecular biology. EMBL research drives the development of new technology and methods in the life sciences. The institute works to transfer this knowledge for the benefit of society.
Fifteen years ago, machine learning and AI were terms familiar mainly to specialised researchers and industry practitioners. Nowadays, AI is a topic for everyone; it’s in the newspapers, and even our dinner conversations turn to it. We’re living in an age when large language models (LLMs) can chat with us and diffusion models can generate […]